Estimating Value-at-Risk in the EURUSD Currency Cross from Implied Volatilities Using Machine Learning Methods and Quantile Regression
Peer reviewed, Journal article
Published version
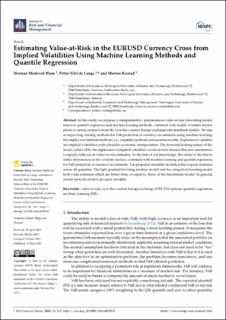
Permanent lenke
https://hdl.handle.net/11250/3109834Utgivelsesdato
2023Metadata
Vis full innførselSamlinger
Originalversjon
10.3390/jrfm16070312Sammendrag
In this study, we propose a semiparametric, parsimonious value-at-risk forecasting model, based on quantile regression and machine learning methods, combined with readily available market prices of option contracts from the over-the-counter foreign exchange rate interbank market. We aim at improving existing methods for VaR prediction of currency investments using machine learning. We employ two different methods, i.e., ensemble methods and neural networks. Explanatory variables are implied volatilities with plausible economic interpretation. The forward-looking nature of the model, achieved by the application of implied volatilities as risk factors, ensures that new information is rapidly reflected in value-at-risk estimates. To the best of our knowledge, this study is the first to utilize information in the volatility surface, combined with machine learning and quantile regression, for VaR prediction of currency investments. The proposed ensemble models achieve good estimates across all quantiles. The light gradient boosting machine model and the categorical boosting model both yield estimates which are better than, or equal to, those of the benchmark model. In general, neural network models are quite unstable.