Deep Reinforcement Learning for Spatio-Temporal Wildlife Management
Master thesis
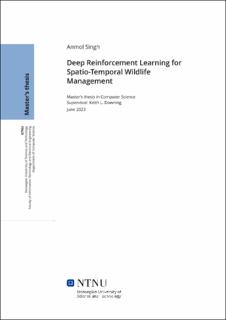
Permanent lenke
https://hdl.handle.net/11250/3097883Utgivelsesdato
2023Metadata
Vis full innførselSamlinger
Sammendrag
De siste 50 årene har globale dyrelivsbestander opplevd betraktelig nedgang som har ført til en biologisk mangfoldskrise hvor et betydelig antall dyrearter har blitt utrydningstruet. Denne oppgaven tester ytelsen til ulike algoritmer av typen dyp forsterkende læring (DRL) innenfor romlig-temporær dyrelivsforvaltning, med formål om å opprettholde biologisk mangfold. DRL er et underområde av maskinlæring som kombinerer dype nevrale nettverk med forsterkende læring, slik at en RL agent kan løse komplekse problemer i avanserte miljøer.
I de siste årene har DRL blitt stadig mer populært grunnet sin vellykkede anvendelse i spill som sjakk og Atari 2600. Det har imidlertid vært få forsøk på å anvende det på dyrelivsforvaltning. Dermed er potensialet til DRL i dette feltet i stor grad uutforsket. For å adressere dette tester denne oppgaven ulike DRL-algoritmer innenfor romlig-temporær dyrelivsforvaltning, med formål om å opprettholde biologisk mangfold. Dette ble gjort ved å lage en romlig-temporær dyrelivsforvaltningssimulator, og trene DRL-algoritmene: DQN, A2C og PPO på den. Fokuset til denne oppgaven var å finne det beste handlingssettet for RL agenten, og å finne DRL-algoritmen med best ytelse. Ytelsen til algoritmene ble basert på størrelsene til artspopulasjonene.
Resultatene viser at det beste handlingssettet består av handlinger som legger til et mindre antall dyr til økosystemet. Selv om alle DRL-algoritmene forbedret seg, tyder resultatene på at det er en avveining mellom ytelse og stabilitet over treningstiden. Ettersom treningstiden øker, skiller PPO seg ut ved at den yter på nivå med DQN og A2C samtidig som den er betydelig mer stabil. Denne oppgaven har vist potensialet til DRL for dyrelivsforvaltning, og framtidig arbeid bør undersøke hvordan andre AI-teknikker, som konvolusjonelle nevrale nettverk og evolusjonsstrategier, kan anvendes i dette domenet. In the past 50 years, global wildlife populations have plummeted resulting in a biodiversity crisis where a significant number of species are at risk of extinction. This project tests the performance of various deep reinforcement learning (DRL) algorithms on the task of spatio-temporal wildlife management, for the purpose of maintaining biodiversity. DRL is a subfield of machine learning that combines deep neural networks with reinforcement learning, thus enabling an RL agent to solve complex problems in intricate environments.
In recent years, DRL has become increasingly popular due to its successful application in games like Chess and Atari 2600. However, there have been limited efforts to apply it to the field of wildlife management. Thus, the potential of DRL in this field remains largely unexplored. To address this, this thesis tests various DRL algorithms on the task of spatio-temporal wildlife management with the purpose of maintaining biodiversity. This was done by creating a spatio-temporal wildlife management simulation and training the DRL algorithms: DQN, A2C, and PPO on it. The focus of this thesis was to find the best action set for the RL agent, and the DRL algorithm with the best performance. The performance of the algorithms was based on the sizes of the species populations.
The results show that the best action set consists of actions that add fewer animals to the ecosystem. While all DRL algorithms were able to improve, the results indicate that there is a trade-off between performance and stability over training time. However, as training time increases PPO stands out as it performs similarly to DQN and A2C while being significantly more stable. This thesis shows the potential of DRL for wildlife management, and future work should investigate the applicability of other AI techniques, such as convolutional neural networks and evolutionary strategies, in this domain.