Efficient prediction of wind and wave induced long-term extreme load effects of floating suspension bridges using artificial neural networks and support vector machines
Journal article, Peer reviewed
Accepted version
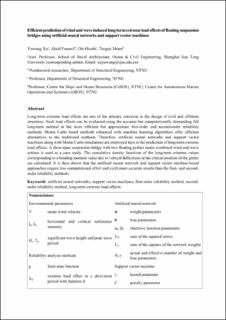
Åpne
Permanent lenke
https://hdl.handle.net/11250/2754188Utgivelsesdato
2020Metadata
Vis full innførselSamlinger
- Institutt for konstruksjonsteknikk [2460]
- Institutt for marin teknikk [3397]
- Publikasjoner fra CRIStin - NTNU [37221]
Sammendrag
Long-term extreme load effects are one of the primary concerns in the design of civil and offshore structures. Such load effects can be evaluated using the accurate but computationally demanding full long-term method or the more efficient but approximate first-order and second-order reliability methods. Monte Carlo based methods enhanced with machine learning algorithms offer efficient alternatives to the traditional methods. Therefore, artificial neural networks and support vector machines are used as surrogate models for the limit state function to speed up the prediction of long-term extreme load effects. A three-span suspension bridge with two floating pylons under combined wind and wave actions is used as a case study. The cumulative density functions of the long-term extreme values corresponding to a bending moment value due to vertical deflections at the critical position of the girder are calculated. It is then shown that the artificial neural network and support vector machine-based approaches require less computational effort and yield more accurate results than the first- and second-order reliability methods.