Approximate Filtering Approaches for Switching Linear Dynamical Systems
Master thesis
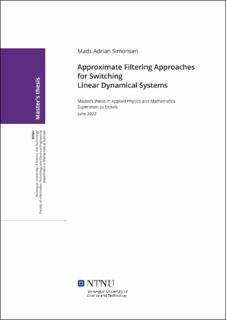
Permanent lenke
https://hdl.handle.net/11250/3027681Utgivelsesdato
2022Metadata
Vis full innførselSamlinger
Sammendrag
«State-observation model» er et dynamisk Bayesiansk nettverk (DBN) som inneholder to mengder stokastiske variabler som utvikler seg over tid; en skjult mengde og en observert mengde.De mest kjente «state-observation» modellene er «hidden Markov model» (HMM) og lineære dynamiske system (LDS), hvor den velkjente Kalmanfilter-metoden er brukt for statistisk inferens.«Switching linear dynamical system» (SLDS) modeller er en utvidelse av LDSer, som inneholder både diskrete og kontinuerlige skjulte variabler, og hvor den observerte variabelen er kontinuerlig.Hovedidéen bak SLDSer er at vi kan bryte ned kompleks dynamisk atferd i mer forståelige stykker som vi kan kategorisere, f.eks. så kan vi kategorisere bevegelsene til et fly inn følgende tilstander: letter, senker og svinger.
Problemet med SLDS er at siden DBNet inneholder både diskrete og kontinuerlige variabler, når vi regner ut posterioirisannsynlighetene gitt observasjonene, en prosess som kalles filtrering, så ender vi opp med en Gaussisk miksfordeling, som øker eksponensielt hvert tidssteg.Vi må derfor søke etter alternative approksimasjoner for filtreringen.
I denne oppgaven, utleder vi det statistiske rammeverket for SLDSer og DBNer generelt, hvor hovedfokuset er filtrering.Deretter sammenligner vi approksimative filtreringsmetoder hvor de deterministiske metodene løser problemet ved å begrense størrelsen til miksen samtidig som vi bevarer de to første momentene, (forventingsverdi og varians), mens den stokastiske metoden løser problemet med en teknikk som kalles partikkelfiltrering («particle filtering»).
Til slutt viser vi en anvendelse av SLDS, hvor målet er å detektere når vi går, og når vi er på bussen, gitt GPS-data (Global Positioning System). The state-observation model is a dynamic Bayesian network (DBN) that contains two set of variables that evolves over time; a hidden set of variables, and an observed set of variables.The most common state-observation models are the hidden Markov model (HMM) and the linear dynamical system (LDS) where the well known Kalman filter is used for inference.Switching linear dynamical system (SLDS) models are an extension of LDSs, that contain both discrete and continuous hidden variables, whereas the observation variable is continuous.The main idea behind SLDSs is that we can break down complex dynamical behavior into more comprehensible pieces.For instance, we can categorize the motions of an aircraft into the states climbing, descending and maneuver.
The problem with SLDS is that due to the DBN containing both discrete and continuous variables, when we compute the posterior probabilities conditioned on the observations, a process called filtering, we end up with a Gaussian mixture which increases exponentially at each time step.Therefore we must look for alternative approximate filtering approaches.
In this thesis we develop the statistical framework for SLDSs and DBNs generally, where the main focus is filtering.We then compare approximate filtering approaches where the deterministic approaches solves the problem by constraining the size of the mixture while preserving the first two moments, (mean and variance), and the stochastic approach solves the problem by particle filtering.Finally we show an application of the SLDS, where the goal is to detect when we are walking, and when we are on the bus given GPS (Global Positioning System) data.