Ensemble updating of binary state vectors by maximising the expected number of unchanged components
Peer reviewed, Journal article
Published version
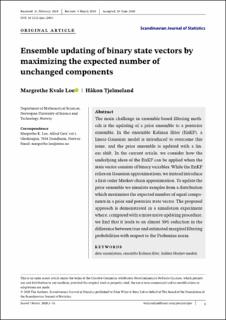
Åpne
Permanent lenke
https://hdl.handle.net/11250/2726846Utgivelsesdato
2020Metadata
Vis full innførselSamlinger
- Institutt for matematiske fag [2353]
- Publikasjoner fra CRIStin - NTNU [37220]
Originalversjon
10.1111/sjos.12483Sammendrag
The main challenge in ensemble-based filtering methods is the updating of a prior ensemble to a posterior ensemble. In the ensemble Kalman filter (EnKF), a linear-Gaussian model is introduced to overcome this issue, and the prior ensemble is updated with a linear shift. In the current article, we consider how the underlying ideas of the EnKF can be applied when the state vector consists of binary variables. While the EnKF relies on Gaussian approximations, we instead introduce a first-order Markov chain approximation. To update the prior ensemble we simulate samples from a distribution which maximizes the expected number of equal components in a prior and posterior state vector. The proposed approach is demonstrated in a simulation experiment where, compared with a more naive updating procedure, we find that it leads to an almost 50% reduction in the difference between true and estimated marginal filtering probabilities with respect to the Frobenius norm.