Diagnosing Highly-Parallel OpenMP Programs with Aggregated Grain Graphs
Journal article, Peer reviewed
Accepted version
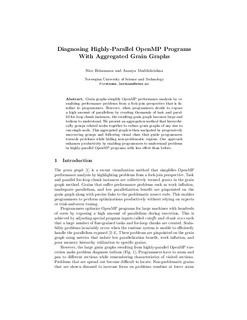
View/ Open
Date
2018Metadata
Show full item recordCollections
Original version
10.1007/978-3-319-96983-1_8Abstract
Grain graphs simplify OpenMP performance analysis by visualizing performance problems from a fork-join perspective that is familiar to programmers. However, when programmers decide to expose a high amount of parallelism by creating thousands of task and parallel for-loop chunk instances, the resulting grain graph becomes large and tedious to understand. We present an aggregation method that hierarchically groups related nodes together to reduce grain graphs of any size to one single node. This aggregated graph is then navigated by progressively uncovering groups and following visual clues that guide programmers towards problems while hiding non-problematic regions. Our approach enhances productivity by enabling programmers to understand problems in highly-parallel OpenMP programs with less effort than before.