Block scheduling at magnetic resonance imaging labs
Gullhav, Anders N.; Christiansen, Marielle; Nygreen, Bjørn; Aarlott, Mats Mathisen; Medhus, Jon Erik; Skomsvoll, Johan Fredrik; Østbyhaug, Per Olav
Journal article, Peer reviewed
Accepted version
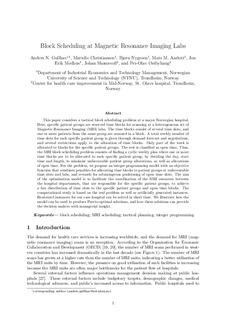
Åpne
Permanent lenke
http://hdl.handle.net/11250/2458193Utgivelsesdato
2017Metadata
Vis full innførselSamlinger
Originalversjon
https://doi.org/10.1016/j.orhc.2017.08.001Sammendrag
This paper considers a tactical block scheduling problem at a major Norwegian hospital. Here, specific patient groups are reserved time blocks for scanning at a heterogeneous set of Magnetic Resonance Imaging (MRI) labs. The time blocks consist of several time slots, and one or more patients from the same group are scanned in a block. A total weekly number of time slots for each specific patient group is given through demand forecast and negotiations, and several restrictions apply to the allocation of time blocks. Only part of the week is allocated to blocks for the specific patient groups. The rest is classified as open time. Thus, the MRI block scheduling problem consists of finding a cyclic weekly plan where one or more time blocks are to be allocated to each specific patient group, by deciding the day, start time and length, to minimise unfavourable patient group allocations, as well as allocations of open time. For the problem, we propose an integer programming model with an objective function that combines penalties for allocating time blocks to patient groups at unfavourable time slots and labs, and rewards for advantageous positioning of open time slots. The aim of the optimisation model is to facilitate the coordination of the MRI resources between the hospital departments, that are responsible for the specific patient groups, to achieve a fair distribution of time slots to the specific patient groups and open time blocks. The computational study is based on the real problem as well as artificially generated instances. Real-sized instances for our case hospital can be solved in short time. We illustrate how the model can be used to produce Pareto optimal solutions, and how these solutions can provide the decision makers with managerial insight.