Fast and accurate group outlier detection for trajectory data
Peer reviewed, Journal article
Accepted version
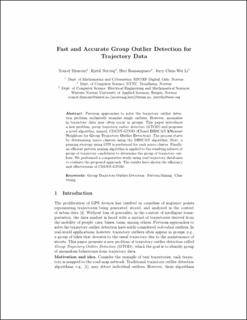
Åpne
Permanent lenke
https://hdl.handle.net/11250/2732233Utgivelsesdato
2020Metadata
Vis full innførselSamlinger
Originalversjon
Communications in Computer and Information Science. 2020, 1259 60-70. 10.1007/978-3-030-54623-6_6Sammendrag
Previous approaches to solve the trajectory outlier detection problem exclusively examine single outliers. However, anomalies in trajectory data may often occur in groups. This paper introduces a new problem, group trajectory outlier detection (GTOD) and proposes a novel algorithm, named, CD kNN -GTOD (Closed DBSCAN kNearest Neighbors for Group Trajectory Outlier Detection). The process starts by determining micro clusters using the DBSCAN algorithm. Next, a pruning strategy using kNN is performed for each micro cluster. Finally, an efficient pattern mining algorithm is applied to the resulting subsets of group of trajectory candidates to determine the group of trajectory outliers. We performed a comparative study using real trajectory databases to evaluate the proposed approach. The results have shown the efficiency and effectiveness of CD kNN -GTOD.