Out-of-the-Box Reproducibility: A Survey of Machine Learning Platforms
Chapter
Accepted version
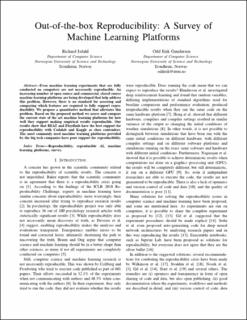
Åpne
Permanent lenke
https://hdl.handle.net/11250/2655335Utgivelsesdato
2019Metadata
Vis full innførselSamlinger
Originalversjon
10.1109/eScience.2019.00017Sammendrag
Even machine learning experiments that are fully conducted on computers are not necessarily reproducible. An increasing number of open source and commercial, closed source machine learning platforms are being developed that help address this problem. However, there is no standard for assessing and comparing which features are required to fully support reproducibility. We propose a quantitative method that alleviates this problem. Based on the proposed method we assess and compare the current state of the art machine learning platforms for how well they support making empirical results reproducible. Our results show that BEAT and Floydhub have the best support for reproducibility with Codalab and Kaggle as close contenders. The most commonly used machine learning platforms provided by the big tech companies have poor support for reproducibility.