A decentralized sensor fusion approach to human fatigue monitoring in maritime operations
Chapter
Accepted version
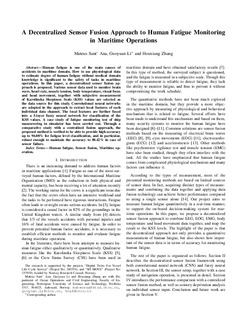
Åpne
Permanent lenke
http://hdl.handle.net/11250/2618814Utgivelsesdato
2019Metadata
Vis full innførselSamlinger
Sammendrag
Human fatigue is one of the main causes of accidents in maritime domain. How to use physiological data to estimate degree of human fatigue without medical domain knowledge is significant to the safety of tasks in maritime operations. In this paper, a decentralized sensor fusion approach is proposed. Various sensor data used to monitor brain wave, heart rate, muscle tension, body temperature, visual focus and head movement, together with subjective measurement of Karolinska Sleepiness Scale (KSS) values are selected as the data source for this study. Convolutional neural networks are adopted in the approach to extract local features of each individual data channel. The local features are further fused into a 5-layer fuzzy neural network for classification of the KSS values. A case study of fatigue monitoring test of ship maneuvering in simulator has been carried out. Through a comparative study with a centralized fusion approach, the proposed method is verified to be able to provide high accuracy up to 96.08% for fatigue level classification, and in particular, robust enough to maintain the accuracy to 88.42% in case of sensor failure.